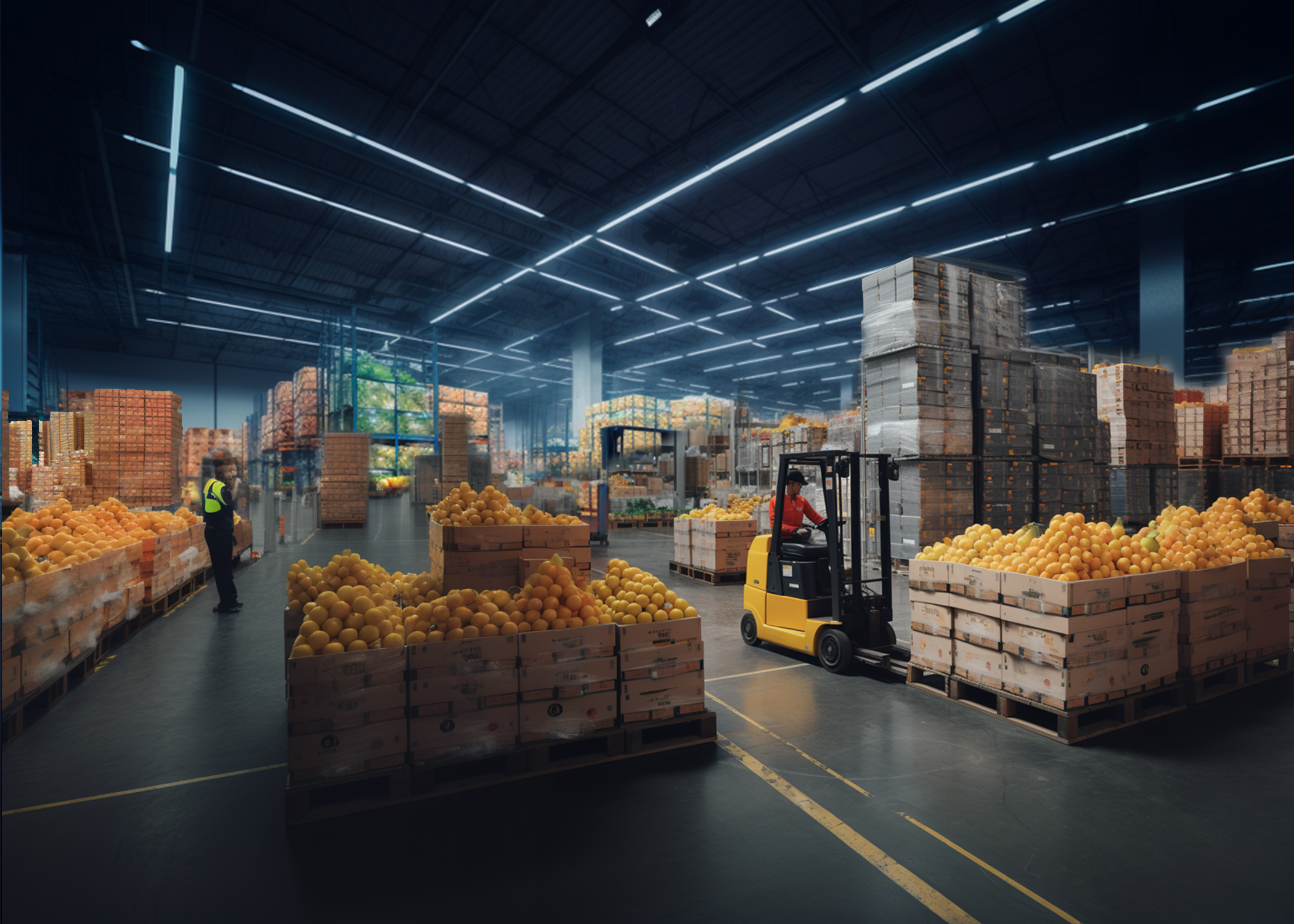
Whether you're an executive, a logistics professional, a data scientist or anything in between, our intuitive solutions will enable you to identify key challenges, optimize processes, and drive real-time results across your organization.
Agentic Applications Designed for your Business
SWARM’s no-code solutions are designed to empower business users – regardless of job function.